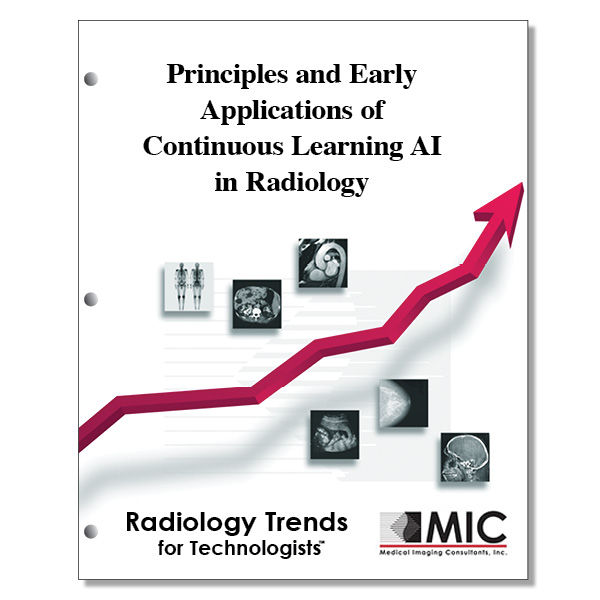
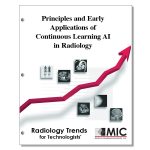
Principles and Early Applications of Continuous Learning AI in Radiology
Deficiencies of artificial intelligence (AI) are presented, and the principle concepts and challenges required for implementing continuous learning AI in radiology are reviewed.
Course ID: Q00657 Category: Radiology Trends for Technologists Modalities: Cardiac Interventional, CT, Mammography, MRI, Nuclear Medicine, Radiation Therapy, Radiography, Sonography, Vascular Interventional2.5 |
Satisfaction Guarantee |
$29.00
- Targeted CE
- Outline
- Objectives
Targeted CE per ARRT’s Discipline, Category, and Subcategory classification:
[Note: Discipline-specific Targeted CE credits may be less than the total Category A credits approved for this course.]
Mammography: 0.75
Image Production: 0.75
Image Acquisition and Quality Assurance: 0.75
Outline
- Introduction
- Key Implementation Principles
- Evolving with the Most Recent Data
- Scaling Up with Broader Data Range and Feature Discovery
- Maintaining High Model Quality
- Improving Computational Performance and Reproducibility
- Regulatory Requirements
- Future-Looking IA
- Conclusion
Objectives
Upon completion of this course, students will:
- know the three predominate domains of AI automation in radiologic imaging workflow
- understand the deficiencies which limit the practical application of AI in radiology practice
- know the changes that continuous learning AI is designed to utilize in the process of updating and improving
- understand how AI models affect healthcare by interacting continuously with their data environments
- be familiar with the general concepts of AI and continuous learning AI
- understand the various learning methods in AI model training
- be familiar with the shared needs of static AI and continuous learning AI
- know the ways in which continuous learning AI models accomplish their goal of achieving the best result through continuous interaction with their changing input data and continuously learning from each change
- know the scenarios in which continuous learning AI can be beneficial
- know why many static AI models for medical imaging are not designed to remain connected to real-time changes in their production environments and are prone to concept drift
- be familiar with the ways in which the data disconnect problem in radiology AI can be overcome
- be familiar with the delay time prediction model example given in the article and what groups informed further model improvements and development
- understand the purpose of the radiologist when implementing feedback loops in continuous learning AI development in diagnostic radiology
- know the factors that AI algorithms transform into predictive model features and, in turn, determine its accuracy
- know the examples listed in the article of diverse source data used for feature engineering to support adaptive learning in diagnostic imaging
- know the benefit of broadening data access and implementing automated feature engineering for continuous learning AI beyond just accuracy improvements
- know what is needed to monitor the quality and consistency of continuous learning AI
- know some examples of disruptions that result in the models demonstrating a completely unintended behavior after retraining
- be familiar with some types of radiology data that are still often collected manually and in an unstructured format
- understand what must be done when developing and retraining continuous learning AI algorithms
- know what is challenging about continuous learning AI having such a rapid evolution
- understand the results from AI quality becoming dependent a specific hardware configuration and software environment
- know what input cannot be simply added to solve the AI performance problem since the effort to find a good solution increases exponentially with the size of the input data and the number of predicted features
- know what is needed for the retraining abilities of new continuous learning AI algorithms due to their requiring relearning at frequent time intervals
- know the essential role of the radiologists’ input for the development and maintenance of the continuous learning AI tools
- be familiar with the good machine learning practices to which AI model developers must adhere to stay within the framework of the scope of continuous learning AI model changes being regulated instead of the changes themselves
- know the necessary components of a change control plan for continuous learning AI
- know how the FDA and the European Commission differ on their approval process for AI software
- understand the ways that continuous learning AI can view processes in progression and overcome the “snapshot” problem of static AI
- know the factors that will impact the future of continuous learning AI